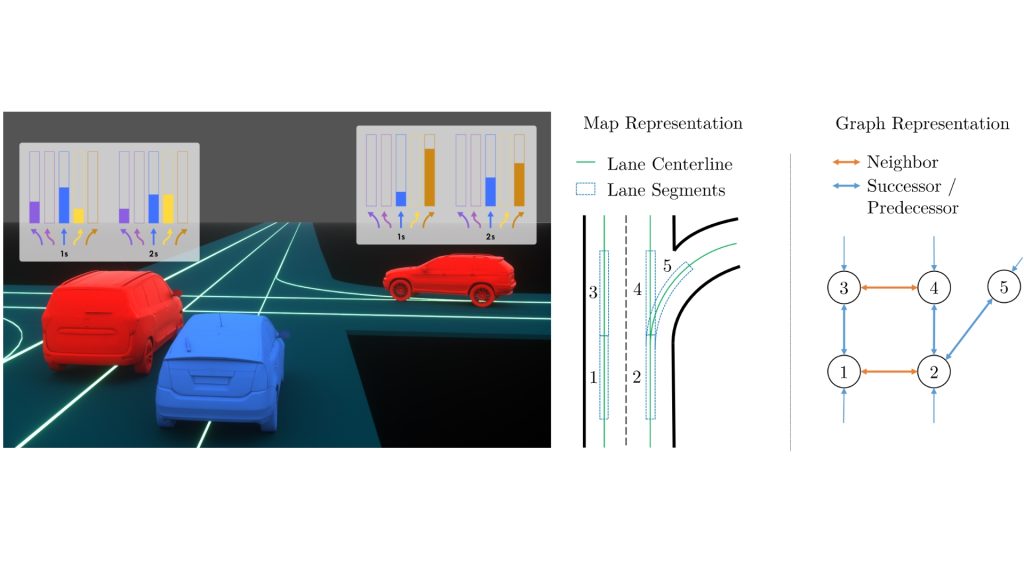
Jan-Nico Zaech, Dengxin Dai, Alexander Liniger, Luc Van Gool
International Conference on Intelligent Robots and Systems, IROS 2020
Abstract
This work studies the problem of predicting the sequence of future actions for surrounding vehicles in real-world driving scenarios. To this aim, we make three main contributions. The first contribution is an automatic method to convert the trajectories recorded in real-world driving scenarios to action sequences with the help of HD maps. The method enables automatic dataset creation for this task from large-scale driving data. Our second contribution lies in applying the method to the well-known traffic agent tracking and prediction dataset Argoverse, resulting in 228,000 action sequences. Additionally, 2,245 action sequences were manually annotated for testing. The third contribution is to propose a novel action sequence prediction method by integrating past positions and velocities of the traffic agents, map information and social context into a single end-to-end trainable neural network. Our experiments prove the merit of the data creation method and the value of the created dataset โ prediction performance improves consistently with the size of the dataset and shows that our action prediction method outperforms comparing models.